Overview of the main principles of Deep Learning along with common architectures. Formulate the problem for time-series classification and apply it to vital signals such as ECG. Applying this methods in Electronic Health Records is challenging due to the missing values and the heterogeneity in EHR, which include both continuous, ordinal and categorical variables. Subsequently, explore imputation techniques and different encoding strategies to address these issues. Apply these approaches to formulate clinical prediction benchmarks derived from information available in MIMIC-III database.

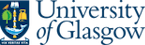
Deep learning in Electronic Health Records - CDSS 2
This course is part of Informed Clinical Decision Making using Deep Learning Specialization
Taught in English

Instructor: Fani Deligianni
Included with
Course
Recommended experience
What you'll learn
Train deep learning architectures such as Multi-layer perceptron, Convolutional Neural Networks and Recurrent Neural Networks for classification
Validate and compare different machine learning algorithms
Preprocess Electronic Health Records and represent them as time-series data
Imputation strategies and data encodings
Skills you'll gain
Details to know

Add to your LinkedIn profile
5 quizzes
Course
Recommended experience
See how employees at top companies are mastering in-demand skills
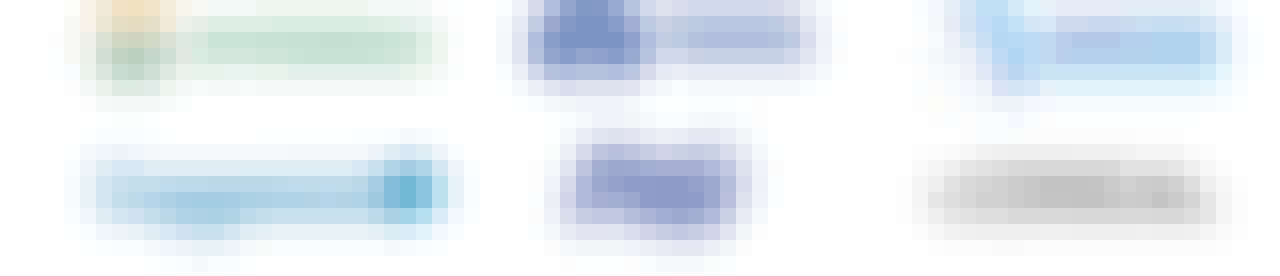
Build your subject-matter expertise
- Learn new concepts from industry experts
- Gain a foundational understanding of a subject or tool
- Develop job-relevant skills with hands-on projects
- Earn a shareable career certificate
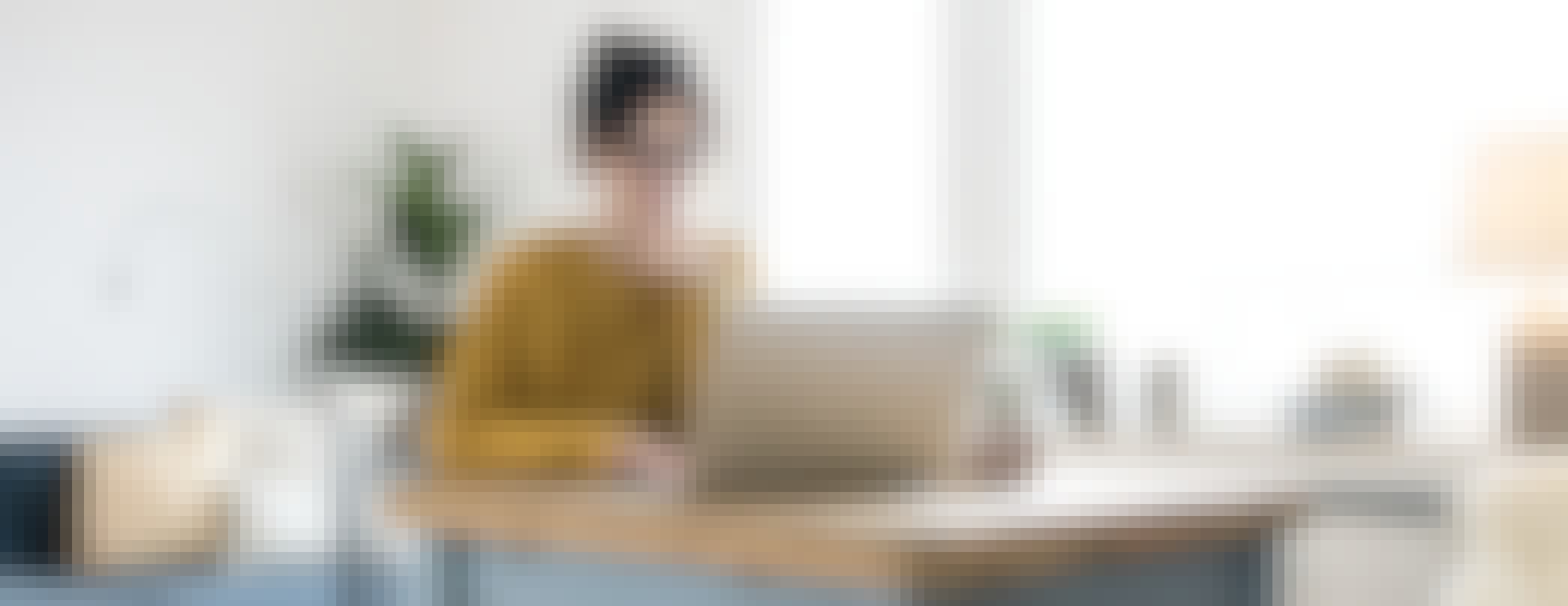
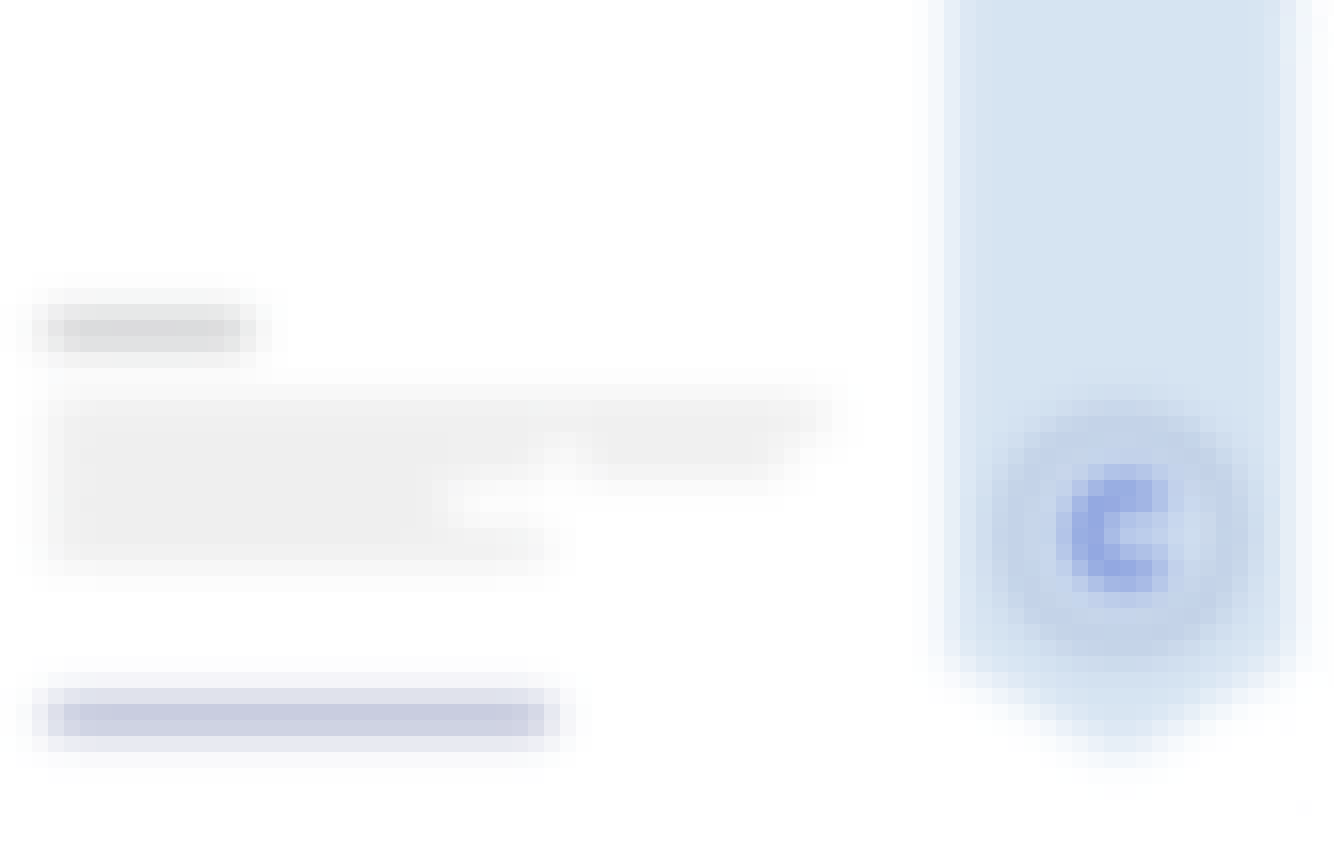
Earn a career certificate
Add this credential to your LinkedIn profile, resume, or CV
Share it on social media and in your performance review
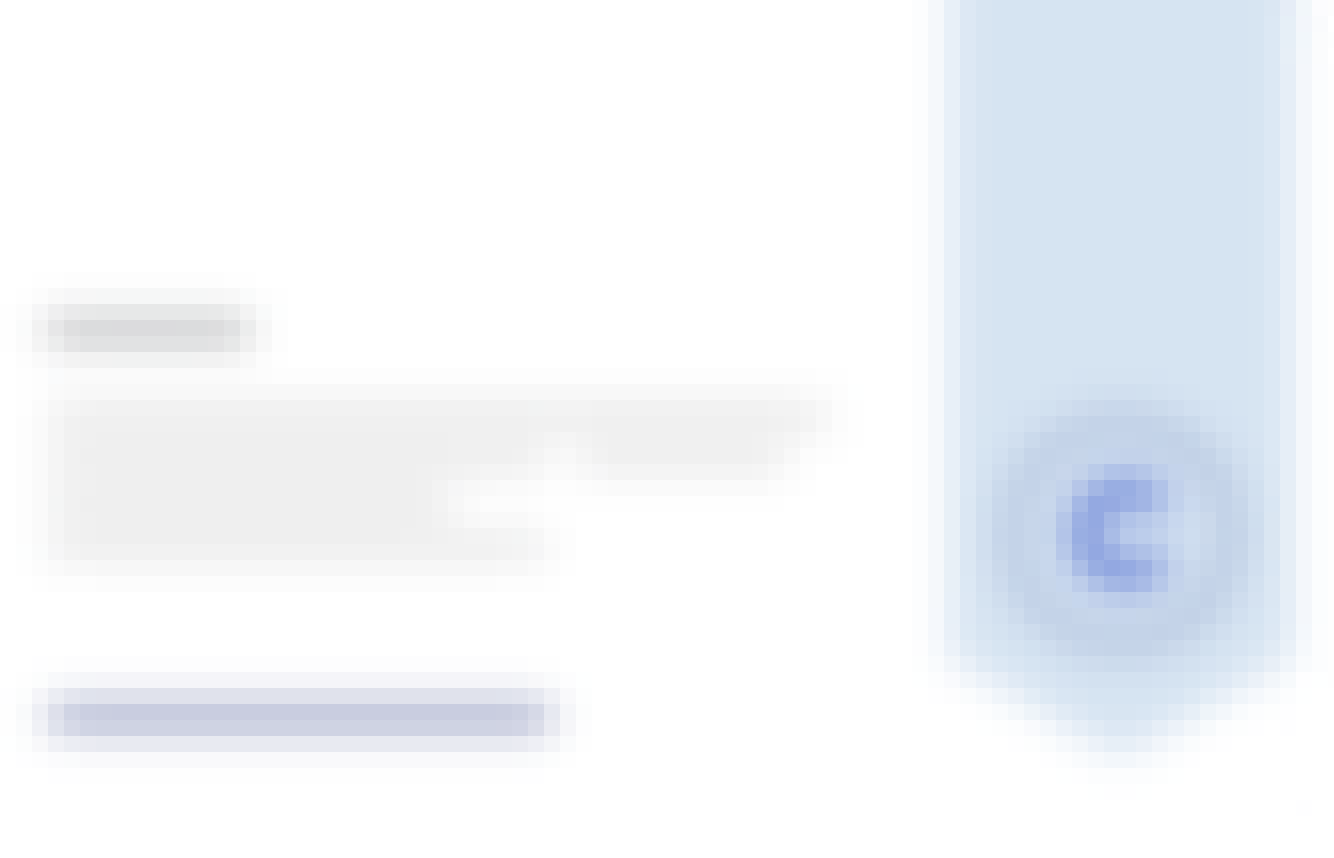
There are 4 modules in this course
This week includes an overview of deep learning history and popular deep learning platforms. Subsequently, Multi-Layer Perceptron (MLP) Networks are discussed along with common activation functions, loss functions and optimisation algorithms. Finally, the practical exercises will allow to optimise and evaluate MLP in ECG classification.
What's included
7 videos5 readings1 quiz1 discussion prompt4 ungraded labs
Convolutional Neural Networks (CNNs) revolutionised the way we process images and they contributed significantly in deep learning success. This week we are going to discuss what advantages CNNs offer over MLP and we will implement CNNs for time-series classifications. Subsequently, we are going to present Recurrent Neural Networks (RNNs). In particular, we are going to discuss Long-Short Term Memory Networks and Gated Recurrent Unit Networks. Practical exercises will allow to design and train all these types of networks in ECG classification. The importance of training, validation and testing datasets will be emphasised for avoiding overfitting and model evaluation.
What's included
3 videos6 readings1 quiz1 discussion prompt5 ungraded labs
Developing benchmark datasets for DNNs based on MIMIC-III database involves several steps that include cohort selection, unit conversion, outlier removal and aggregation of data within time windows. The later step allows to represent EHR as time-series data but it is also susceptible to missing data. For this reason imputation strategies both based on traditional and deep learning techniques are presented. The learner will have the opportunity to preprocess EHR and train deep learning models in predicting in-hospital mortality.
What's included
4 videos8 readings1 quiz1 discussion prompt5 ungraded labs
EHRs include categorical, ordinal and continuous variables. Appropriate data representation is important and encodings affect prediction performance. This week includes several different strategies to encode the data such as target encodings, deep learning encodings and similarity encodings. In particular, autoencoders which is a deep learning architecture to represent data in lower dimensional space will be demonstrated and applied in in-hospital mortality prediction.
What's included
4 videos5 readings2 quizzes1 discussion prompt4 ungraded labs
Instructor

Offered by
Recommended if you're interested in Machine Learning
University of Glasgow
University of Glasgow
University of Glasgow
University of Glasgow
Why people choose Coursera for their career




New to Machine Learning? Start here.

Open new doors with Coursera Plus
Unlimited access to 7,000+ world-class courses, hands-on projects, and job-ready certificate programs - all included in your subscription
Advance your career with an online degree
Earn a degree from world-class universities - 100% online
Join over 3,400 global companies that choose Coursera for Business
Upskill your employees to excel in the digital economy
Frequently asked questions
Access to lectures and assignments depends on your type of enrollment. If you take a course in audit mode, you will be able to see most course materials for free. To access graded assignments and to earn a Certificate, you will need to purchase the Certificate experience, during or after your audit. If you don't see the audit option:
The course may not offer an audit option. You can try a Free Trial instead, or apply for Financial Aid.
The course may offer 'Full Course, No Certificate' instead. This option lets you see all course materials, submit required assessments, and get a final grade. This also means that you will not be able to purchase a Certificate experience.
When you enroll in the course, you get access to all of the courses in the Specialization, and you earn a certificate when you complete the work. Your electronic Certificate will be added to your Accomplishments page - from there, you can print your Certificate or add it to your LinkedIn profile. If you only want to read and view the course content, you can audit the course for free.
If you subscribed, you get a 7-day free trial during which you can cancel at no penalty. After that, we don’t give refunds, but you can cancel your subscription at any time. See our full refund policy.